Data-Driven Decision-Making: How BRAC Mines Answers From Metrics
If you ever needed proof that data-driven decision-making is crucial to improving business performance, consider this finding from a 2012 Harvard Business Review study of 646 executives, managers and professionals across multiple industries and geographies:
“More than 70 percent of the organizations that had deployed analytics throughout their organizations reported improved financial performance, increased productivity, reduced risks, and faster decision making. Organizations with less widespread distribution of analytics access were typically 20 percentage points less likely to report such benefits.” (Read More)
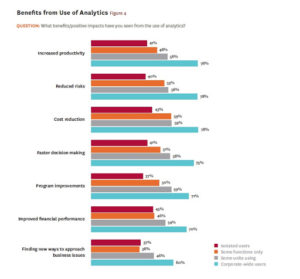
Source: Harvard Business Review Analytic Services Report
Creating a Data-Driven Decision-Making Culture
At BRAC’s Skills Development Programme (SDP) our goal is to enable disadvantaged women and men to have better jobs by improving their skills, their income and their workplaces. To help further those efforts we wanted to not only use data for reporting on what’s already happened but to make decisions for continual improvement – like dispelling inaccurate hypotheses and answering thorny questions that could be inhibiting our program’s success. This meant that we had to develop better data systems and also spark curiosity within our field teams so they would engage with the data and share their insights from the frontlines. (Read More)
On the system front, we’ve used TaroWorks’ mobile offline field service app, the Salesforce cloud database and a range of other analytics tools and techniques to delve into data in a way that is helping our organization foster data-driven decision making.
To build our interest in data, we started a quarterly discussion seminar called Data Talks where, as a team, we would take a deep dive into issues and questions and figure out how to answer the questions with data. These Data Talks have unearthed questions from the field and allowed us to put in place data-driven decision-making. Here are three examples of how we’ve mined answers from our metrics to make decisions.
Solving a Microfinance Mystery
- Background: Promotion Incubation Support to Enterprises (PROMISE) is a BRAC project that supports young entrepreneurs in Bangladesh to successfully develop their businesses and create decent employment opportunities through the establishment of youth-led enterprises in local communities. PROMISE has a loan facility where it helps participants in the program obtain microfinance loans.
- Issue: Many program participants were being turned down for the microfinance loans.
- Data Analysis: BRAC analyzed data associated with program participants who were approved for loans and found these common data points among successful loan applicants:
- Monthly income of more than 8,000 Bangladeshi Taka
- Four or more years of business experience
- Family assets of 500,000 or more Bangladeshi Taka
- No previous loan received
- No previous assistance from the Skills Development Programme
- Result: SDP identified an important mismatch between requirements for entering the overall PROMISE program and requirements for being approved for a microfinance loan, something SDP believed could be hurting loan applicant’s chances. While SDP mandated that PROMISE program participants have 2 or more years of business experience, 4 or more years of such experience was required for receiving microfinance loans. As a result, SDP is considering revising its PROMISE program selection criteria so that selected program participants have a better chance of receiving loans for their businesses. (Read More)
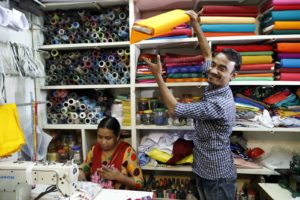
Image: Small business owner in Dhaka suburb. Source: BRAC/Noor Gelal
X Marks the Spot
- Background: BRAC’s Pro-poor growth of rural enterprises through sustainable development (PROGRESS) project works to “catalyse the development of micro and cottage enterprises in the light engineering sector” in Bangladesh. The program supports light engineering company owners (LEOs) to strengthen their business skills. It also places a young person (a “learner”) within the light engineering company as an apprentice who learns valuable engineering skills for future employment in sectors like steel cutting and fabrication, welding, air conditioning and refrigeration, vehicle maintenance, electrical motor rewiring and certain machinist trades.
- Issue: One challenge for the PROGRESS initiative was effectively and efficiently managing the recruiting process used to identify apprentices to be matched with LEOs. In particular, the data-driven decision-making question was: How far from the light engineering facility’s location should the program’s recruiters search for apprentices so as to find the best talent but not make their commute to work onerous?
- Data Analysis: BRAC used GPS coordinates to determine an optimal distance from the potential training site (factory) that someone selected to be trained at that factory should live – based on past apprentice selection data trends. When this data was represented in a cumulative frequency distribution, it was determined that roughly 75% of all the selected learners were within 2.5 kilometers (approximately 1.5 miles) of their LEO’s factories and 90% were within 4.5 kilometers (approximately 2.8 miles).
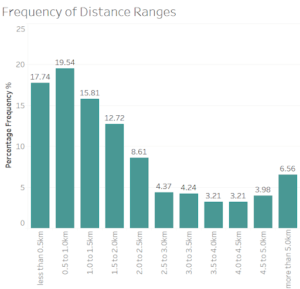
Source: BRAC
- Result: This data-driven decision-making insight was important to ensure that apprentice recruiters didn’t spend their time and energy reaching out to learners who have little chance of being selected for the project given how far away they live from the light engineering operation. BRAC concluded that if surveying is focused on this 4.5 kilometer range, a better quality of potentially selectable learners can be achieved. Secondly, it highlighted the need for the talent recruiters to know the location of the nearest LEOs rather than basing their surveying for potential apprentices around the nearest BRAC branch office – as was the norm. (Read More)
Proxies for Female Participation
- Background: The light engineering sector in Bangladesh is male-dominated and one of the objectives of BRAC’s PROGRESS program is to reach a 40% female participation rate among the learners matched with owners of light engineering manufacturers. So far the best result has been 28%.
- Issue: Planning for program expansion, BRAC needed to get as accurate a data-driven decision-making assessment as possible on whether new light engineering company owners would be willing to take on a woman as an apprentice. A survey of 7000 LEOs had a question regarding whether the LEOs were willing to take in a female learner. We saw a baseline acceptance rate of 64.85% across all the LEOs surveyed. This was much higher than the target 40%. However, this statistic might be misleading because BRAC’s reputation for helping women precedes it – and anyone trying to find their way into this project might reply in the affirmative to this question. So we expected the amount of LEOs who actually would be okay with a female learner to be lower than 64.85%. The problem with using that data point alone is that many LEOs who in-fact would not be comfortable with female learners would hide that fact given the nature of the survey. So we looked for proxies that could approximate a LEO’s likelihood to accept a female learner.
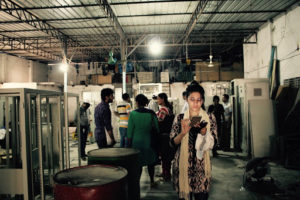
Image: Light engineering factory in Tongi. Source: BRAC/Farzana Trina Chowdhury
- Data Analysis: Examining the data we identified a few potential proxies but only one was deemed reliable:
- Toilet Accessibility: One of the most commonly held beliefs in the PROGRESS program had been that access to a toilet in many of these light engineering factories was bad and that this issue had been a huge barrier to female learner placement. However, looking at the data we determined that regardless of the toilet situation, the percentage of learners placed who were female did not vary much from the baseline – ruling out this factor as a proxy. Despite the lack of variation, BRAC will continue to track this metric to avoid placing female learners with LEOs with no consideration of the toilet situation as this is something that can acutely affect the learner’s training experience.
- Business Experience: The second metric investigated was the popular belief that the more business experience a LEO had, the more likely they were to rise above discriminatory mindsets and realize that there is no difference between male and female workers in their factories. This is a questionable belief as by and large there is no female presence in the light engineering sector in Bangladesh. Our data-driven decision-making findings concurred with this idea – showing that no matter the years of business experience the LEO had, their likelihood to accept female learners did not vary much beyond the baseline acceptance rate from the factory owner survey – disqualifying ing this metric as a proxy.
- Education Level: The final metric investigated was the education level attained by each LEO – again due to the common belief that the more educated you are, , the more accepting willing you will be of to take on female learners. The data showed an interesting insight that can come from data-driven decision-making in that it did not matter how educated the company owner was so long as they attended school for some period of time. From class 1 all the way through to bachelors level in the Bangladeshi school system, the female acceptance rate among LEOs varied very little from the 64.85% baseline acceptance rate in the survey. However, if the LEO had never gone to school, then the acceptance rate dropped to 44.23%. This could be because of a lack of education or that not being sent to primary school as a child was a proxy for another impediment to accepting female learners, like a family who didn’t send their child to school because they had particular views on a woman’s place in the workforce. (Read More)
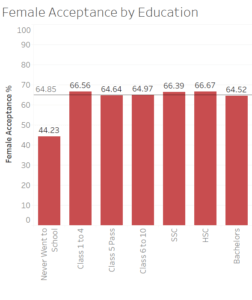
Source: BRAC
- Result: We now consider whether or not the LEO went to school as a good proxy for the likelihood they will accept female learners as apprentices at their factories.
Want To Demo TaroWorks?
Many TaroWorks customers previously used paper and spreadsheets to collect data, analyze metrics and run field operations. Let us show you how TaroWorks’ offline mobile field service app can help scale your business by digitizing sales and supply chain management, increasing agent network productivity and analyzing data in real-time for business insights.
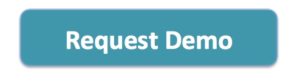
TaroWorks, a Grameen Foundation company.
Site by V+V